この記事では,研究のサーベイをまとめていきたいと思います。ただし,全ての論文が網羅されている訳ではありません。また,分かりやすいように多少意訳した部分もあります。ですので,参考程度におさめていただければ幸いです。
間違えている箇所がございましたらご指摘ください。随時更新予定です。他のサーベイまとめ記事はコチラのページをご覧ください。
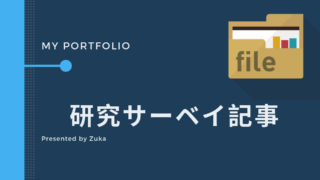
【まとめページ】研究サーベイ記事一覧
和訳記事
●Attentionを用いたseq2seqのメカニズム●イラストでみるTransfomer
機械学習
基本...
スポンサーリンク
本論文を一枚の画像で
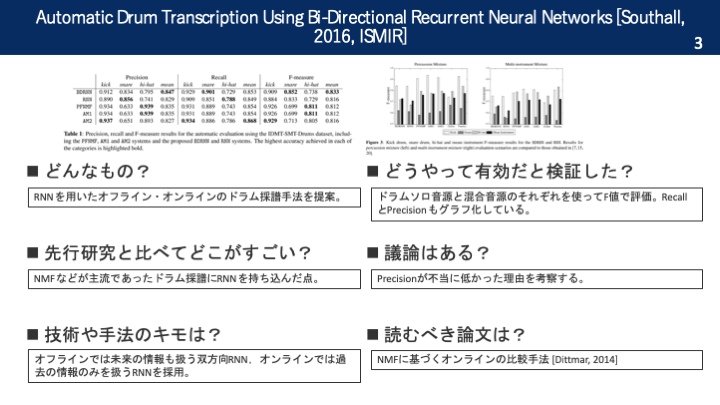
要旨
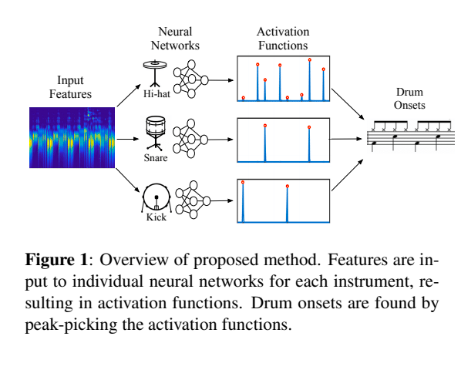
ニューラルネットという強力な手法をドラム採譜に生かす試み。双方向RNNとonlineで利用できるネットワークの2つを提示する。
提案手法
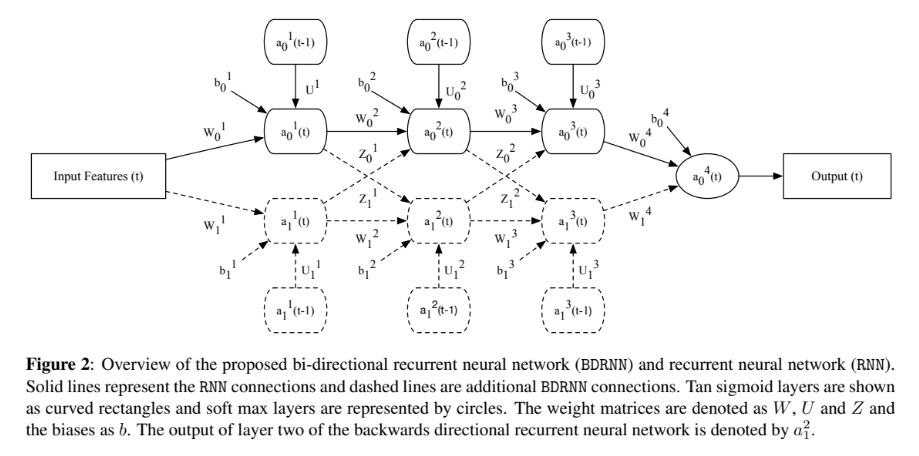
オフライン用に双方向RNN,オンライン用にRNNを提案する。パラメータは事前に実験を行なった結果最適と思われる値を設定している。閾値はアクティベーションの平均値の何倍かで決める。オンセットの間隔は最低50msとしている。
実験・評価
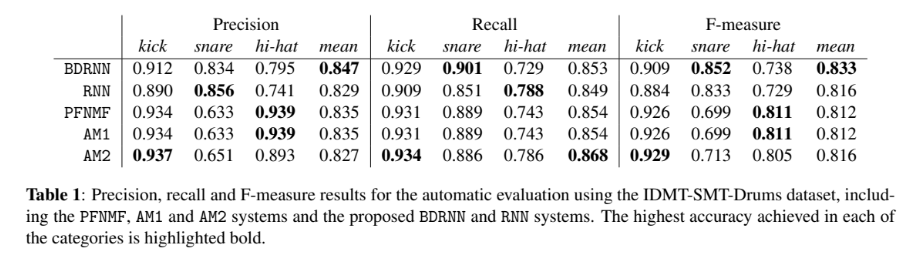
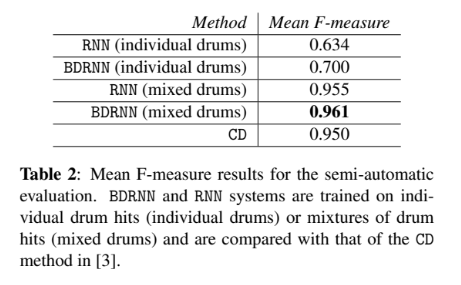
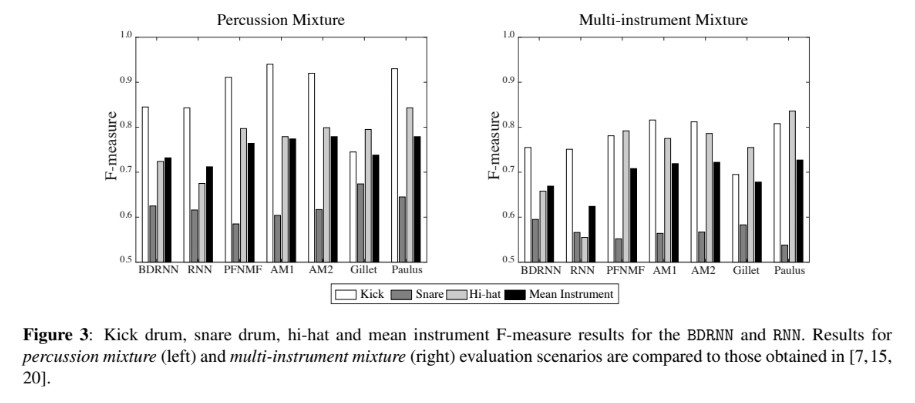
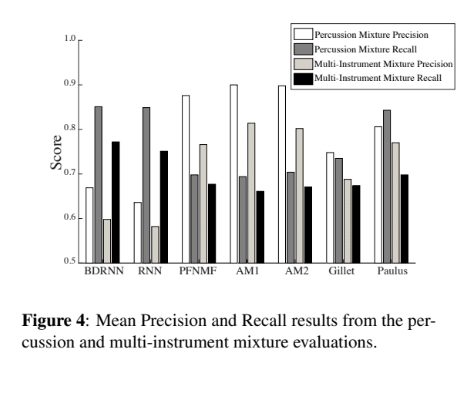
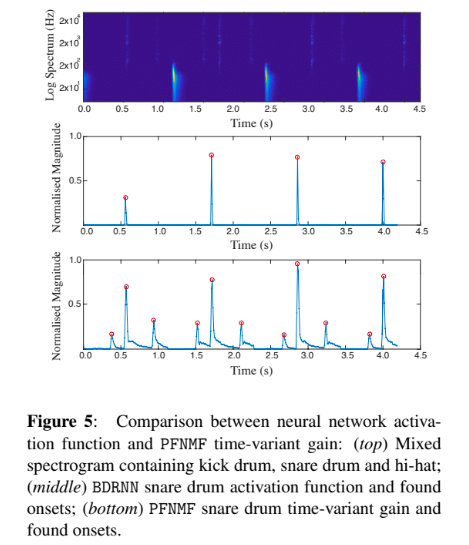
結論
ソロ音源では最高性能を達成。混合音源では何らかの要因によりうまく性能を発揮できなかった。その根拠としては,Recallは高く,Precisionが不当に低いことが挙げられる。また,提案手法にはアクティベーションを鋭くする効能もある。
まとめ
RNNを利用したオフライン・オンラインのドラム採譜手法でした。
参考文献
Southall, Carl, Ryan Stables, and Jason Hockman. “Automatic Drum Transcription Using Bi-Directional Recurrent Neural Networks.” ISMIR. 2016.